Laboratory
The ODISSEI Laboratory develops the innovations that will shape the infrastructure for the future and ensures that new forms of data can be analysed by researchers.
It is a work stream in the ODISSEI Roadmap project. The other work streams are the Data Facility, Observatory, and Hub.
The Laboratory consists of five closely interrelated tasks:
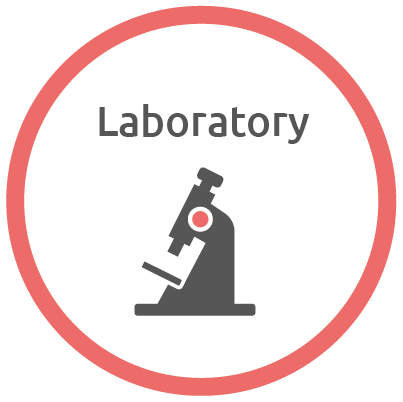
3.1 Distributed analytics techniques
ODISSEI will seek to examine the potential for application in the social sciences through the use of distributed computation through federated learning algorithms. This has been applied in the Personal Health Train which is a concept that was developed in the medical sciences. In the Verantwoordelijke Waardecreatie met Big Data project, funded by the NWA (project number: 400.17.605), Maastricht University together with The Maastricht Study and Statistics Netherlands are implementing the Personal Health Train on health data. Its basic concept is that several stakeholders want to collaborate in data analysis, but do not want or are legally not allowed to share the data with one another – often due to privacy considerations. Rather than appointing a Trusted Third Party, the researchers develop an algorithm (in this analogy: the train) that visits each stakeholder (‘station’), analyses the data on site and goes to the next station with the analysis results, but without the data. The concept of distributed computation has been widely studied in the medical and computer sciences (Sun et al., 2018). Though having great potential in the social sciences, to date, no research has been done about its applications in this field. ODISSEI will examine the possibilities for use of distributed analytics techniques in the social sciences. For example, educational data which is highly federated and stored securely in hundreds of individual schools might be analysed without the need to collate and centralize data. The use of such an approach is not without risks and extensive testing and prototyping will be required. Other ODISSEI data collections with access and technical restrictions within the ODISSEI data facility architecture will also be identified and the potential of distributed computing will be examined along with potential data providers in collaboration with the Data Scout team in the Observatory.
Project team Distributed analysis techniques: Michel Dumontier (Maastricht University – Task leader).
Questions regarding Distributed analysis techniques? Contact Lucas van der Meer (ODISSEI Coordination Team).
3.2 Automated data linkage
A promising, though high-risk area, in the field of computer science is automated data linkage. This has the potential of strongly reducing the time needed by researchers to manually link datasets while performing analysis. Linking datasets is one of ODISSEI’s core aims. The social data within ODISSEI is highly structured and persistent identifiers and standardised codes are used pervasively. However, the degree of automated linkage is restricted by the lack of infrastructure that exists for such linkage. Computer science techniques are advancing through the use of deep learning methods which help identify and link more opaque constructs within data that are less well defined and structured such as families, social networks, neighbourhoods or even cultural groups. The potential of such techniques in the social sciences is considerable but these approaches require high levels of expertise. In this subtask, these high risk/high reward approaches will be explored and examined to see if they can complement the more functional and established manual and semi-automated linkages which will be made in the development of the ODISSEI Portal. Work will start with the construction of a knowledge graph of ODISSEI data collections and data sources in order to assess the scope and potential for automated linkage.
Project team Distributed analysis techniques: Jacco van Ossenbruggen (VU Amsterdam – Task leader).
Questions regarding Automated data linkage? Contact Lucas van der Meer (ODISSEI Coordination Team).
3.3 Innovative Survey Experiments
ODISSEI will strengthen and develop the LISS panel in several ways. Firstly, ODISSEI will provide an upgrade of the existing LISS panel through a panel refreshment of a further 1,000 households in the first, third, and fifth year of the project. This will increase the representativity of LISS and capture subpopulations that the current panel is not adequately resourced to capture. Secondly, ODISSEI will provide extensive space on the LISS panel for researchers at ODISSEI member organisations for the deployment of their own research designs and experiments.
This recently launched initiative is a hugely successful and inspiring element of ODISSEI. Researchers of any seniority can design their own survey research that is then deployed by the LISS technical team at CentERdata at no cost. These research projects are selected through an open call administered by the ODISSEI Coordination Team. Within the project, ODISSEI will grant space on LISS to between five to ten projects per year via open calls and will evaluate projects on the degree to which they integrate with and enhance the wider ODISSEI infrastructure through data linkage and enrichment. All collected data will be available to the entire academic community at no cost.
Project team Innovative Survey Experiments: Marcel Das (CentERdata – Task leader).
Questions regarding Innovative Survey Experiments? Contact Kasia Karpinska(ODISSEI Coordination Team).
3.4 Mass Experiment Online Lab
The Mass Experiment Online Lab will facilitate experiments in which large numbers of subjects simultaneously interact under controlled conditions. These population-level experiments cannot be conducted in the traditional laboratory as they require scale in which network structure is systematically varied across multiple large-sized experimental populations (Centola, 2007; Bail, Merhout & Ding, 2018). The Mass Experiment Online Lab overcomes (1) the significant infrastructural and logistic challenges associated with simultaneous networked participation of many subjects (Salganik, 2018) and (2) removes barriers-to-entry by providing methodological and organisational research facilities to interested but otherwise ill-equipped domain experts through a series of open calls. The Lab will be piloted iteratively using an agile model, with increasing functionality to service a gradually expanding community of beta users. Data generated will be archived, findable via the ODISSEI Portal and linkable within the ODISSEI Secure Supercomputer.
Project team Mass Experiment Online Lab: Arnout van de Rijt (Utrecht University – Task leader).
Questions regarding Mass Experiment Online Lab? Contact Tom Emery (ODISSEI Coordination Team).
3.5 Citizen Science Platform
The ODISSEI Citizen Science Platform will improve data quality in citizen science by applying existing expertise from the field of social science methodology. Citizen science projects include those which rely on ordinary citizens to collect scientific data at a large scale on for example air pollution, the backyard bird count, or the history of marriage. Underestimated aspects of data collection through citizen science are issues of selectivity and measurement error. For example, what type of citizen participates in science, and does that affect the conclusions? How reliable are the measures collected, and is it possible to estimate the measurement errors and correct for any detrimental effect they might have? How does one make sure that member organisations collect data in such a way as to minimise these errors? Social methodologists are used to developing solutions to such questions for social data collection at the service of social science. ODISSEI will pilot a platform for the benefit of all fields of science that use citizens as data collectors. This platform will perform three functions: (1) trusted and convenient data collection interface for fast development of Citizen Science applications through a web-based and mobile app platform to facilitate data collection; (2) link to spatial and demographic information from Statistics Netherlands to allow post-stratification to adjust for selectivity of the citizen scientist population and investigate the sensitivity of the conclusions; (3) allow double-coding and validation so researchers can estimate and correct for classification errors. The platform will be built open-source and will be modular so that teams can easily add components to the study or change the look-and-feel. Existing components will be recycled as much as possible. Over the project, the ODISSEI Citizen Science Platform will collaborate with existing citizen science projects and invite groups to use the tools and receive support through open calls.
Project team Citizen Science Platform: Peter Lugtig (Utrecht University – Task leader).
Questions regarding Citizen Science Platform? Contact Tom Emery (ODISSEI Coordination Team).